Showing 16225–16236 of 18767 results
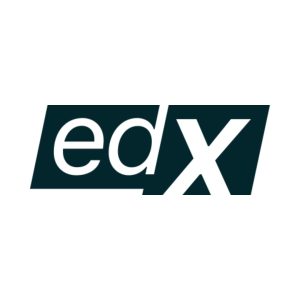
Statistics.comX: Applied Data Science Ethics
AI’s popularity has resulted in numerous well-publicized cases of bias, injustice, and discrimination. Often these harms occur in machine learning projects that have the best of goals, developed by data scientists with good intentions. This course, the second in the data science ethics program for both practitioners and managers, provides guidance and practical tools to build better models and avoid these problems.
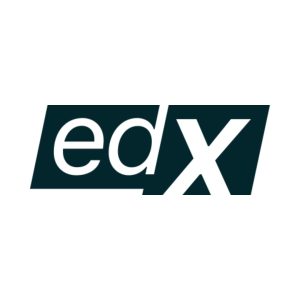
Statistics.comX: Applied Data Science Ethics
AI’s popularity has resulted in numerous well-publicized cases of bias, injustice, and discrimination. Often these harms occur in machine learning projects that have the best of goals, developed by data scientists with good intentions. This course, the second in the data science ethics program for both practitioners and managers, provides guidance and practical tools to build better models and avoid these problems.
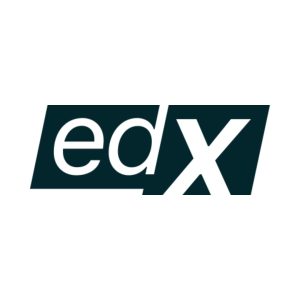
Statistics.comX: Applied Data Science Ethics
AI’s popularity has resulted in numerous well-publicized cases of bias, injustice, and discrimination. Often these harms occur in machine learning projects that have the best of goals, developed by data scientists with good intentions. This course, the second in the data science ethics program for both practitioners and managers, provides guidance and practical tools to build better models and avoid these problems.
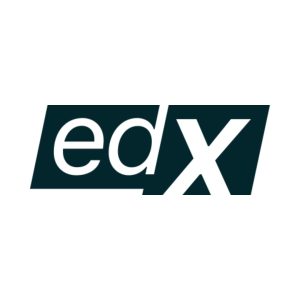
Statistics.comX: Applied Data Science Ethics
AI’s popularity has resulted in numerous well-publicized cases of bias, injustice, and discrimination. Often these harms occur in machine learning projects that have the best of goals, developed by data scientists with good intentions. This course, the second in the data science ethics program for both practitioners and managers, provides guidance and practical tools to build better models and avoid these problems.
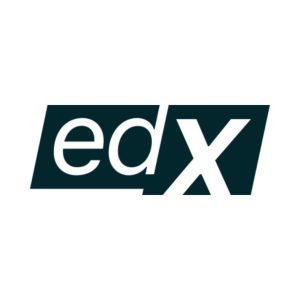
Statistics.comX: Applied Data Science Ethics
AI’s popularity has resulted in numerous well-publicized cases of bias, injustice, and discrimination. Often these harms occur in machine learning projects that have the best of goals, developed by data scientists with good intentions. This course, the second in the data science ethics program for both practitioners and managers, provides guidance and practical tools to build better models and avoid these problems.
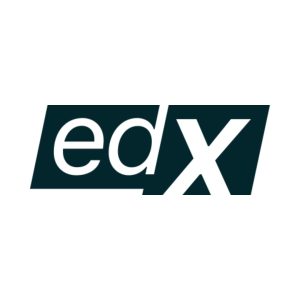
Statistics.comX: MLOps1 (AWS): Deploying AI & ML Models in Production using Amazon Web Services
Most data science projects fail. There are various reasons why, but one of the primary reasons is the challenge of deployment. One piece to the deployment puzzle is understanding how data engineers can effectively work with data scientists to monitor and iterate on model performance, which is why we developed this course: MLOps1 (AWS) - Deploying AI & ML Models in Production using Amazon Web Services.
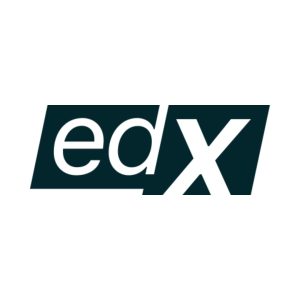
Statistics.comX: MLOps1 (AWS): Deploying AI & ML Models in Production using Amazon Web Services
Most data science projects fail. There are various reasons why, but one of the primary reasons is the challenge of deployment. One piece to the deployment puzzle is understanding how data engineers can effectively work with data scientists to monitor and iterate on model performance, which is why we developed this course: MLOps1 (AWS) - Deploying AI & ML Models in Production using Amazon Web Services.
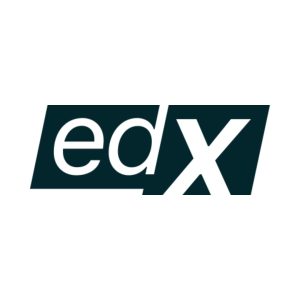
Statistics.comX: MLOps1 (AWS): Deploying AI & ML Models in Production using Amazon Web Services
Most data science projects fail. There are various reasons why, but one of the primary reasons is the challenge of deployment. One piece to the deployment puzzle is understanding how data engineers can effectively work with data scientists to monitor and iterate on model performance, which is why we developed this course: MLOps1 (AWS) - Deploying AI & ML Models in Production using Amazon Web Services.
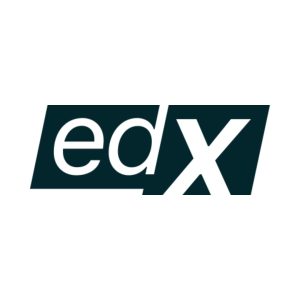
Statistics.comX: MLOps1 (Azure): Deploying AI & ML Models in Production using Microsoft Azure Machine Learning
Most data science projects fail. There are various reasons why, but one of the primary reasons is the challenge of deployment. One piece to the deployment puzzle is understanding how data engineers can effectively work with data scientists to monitor and iterate on model performance, which is why we developed this course: MLOps1 (Azure) - Deploying AI & ML Models in Production using Microsoft Azure Machine Learning
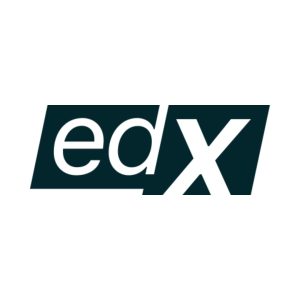
Statistics.comX: MLOps1 (Azure): Deploying AI & ML Models in Production using Microsoft Azure Machine Learning
Most data science projects fail. There are various reasons why, but one of the primary reasons is the challenge of deployment. One piece to the deployment puzzle is understanding how data engineers can effectively work with data scientists to monitor and iterate on model performance, which is why we developed this course: MLOps1 (Azure) - Deploying AI & ML Models in Production using Microsoft Azure Machine Learning
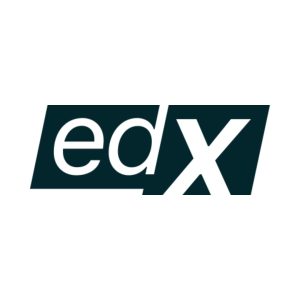
Statistics.comX: MLOps1 (GCP): Deploying AI & ML Models in Production using Google Cloud Platform
Most data science projects fail. There are various reasons why, but one of the primary reasons is the challenge of deployment. One piece to the deployment puzzle is understanding how data engineers can effectively work with data scientists to monitor and iterate on model performance, which is why we developed this course: MLOps1 (GCP) - Deploying AI & ML Models in Production using Google Cloud Platform
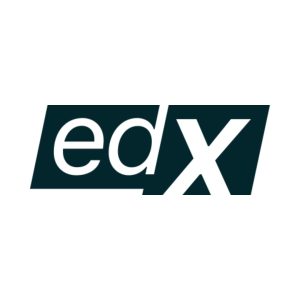
Statistics.comX: MLOps1 (GCP): Deploying AI & ML Models in Production using Google Cloud Platform
Most data science projects fail. There are various reasons why, but one of the primary reasons is the challenge of deployment. One piece to the deployment puzzle is understanding how data engineers can effectively work with data scientists to monitor and iterate on model performance, which is why we developed this course: MLOps1 (GCP) - Deploying AI & ML Models in Production using Google Cloud Platform